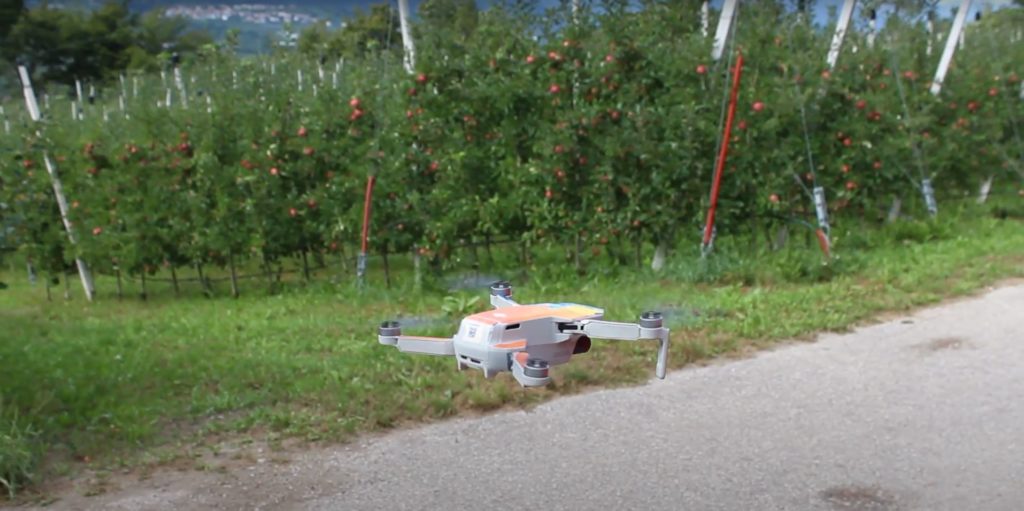
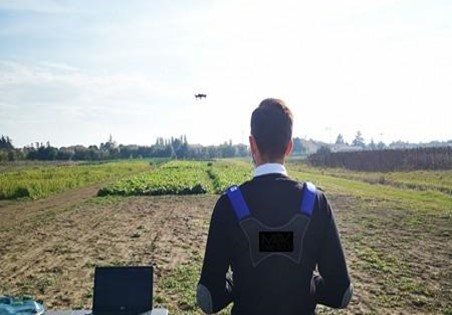
International Cooperation Project: DeepFarm
Deep learning methods have gained the attention of many precision agriculture and remote sensing applications. Accurately mapping agricultural fields is a prerequisite for proper management and the correct yield prediction. Deep learning can provide an effective alternative in detecting, locating, counting, and segmenting trees and plantations. However, a current problem concerning these methods is dealing with high-density object scenes. In this project, we propose to investigate the current state-of-the-art and propose deep learning-based approaches like Convolutional Neural Networks (CNN) to deal with high-density orchard plantation scenes in Unmanned Aerial Vehicle (UAV)-based imagery. The contribution of this study is three-folded:
1) To improve high-density object detection accuracy for agricultural fields
2) To develop a method that simultaneously detects planting row and individual plants
3) To perform semantic segmentation of tree canopies.
We will adopt available data sets acquired on different orchards and crops such as citrus, apple, sugarcane, and soybean, with individual plants identified as point-feature, planting rows as line-feature, and tree canopies as polygon-feature. Our approach will rely on pre-existing methods or networks in current development. An in-development method of ours implements a Pyramid Pooling Module (PPM) and a Multi-Stage Refinement of the confidence map for the detection of the plants’ position through peaks in the confidence map. This approach will be used for locating and counting individuals, as well as for detecting planting rows. As for the segmentation of tree canopies, we intend to develop a semantic classification based on supervised machine-learning networks. We think the results of this joint project could enhance decision farming tasks while contributing to research regarding remote sensing technologies and precision agriculture practices in Brazil and Italy.
Partners
Funders
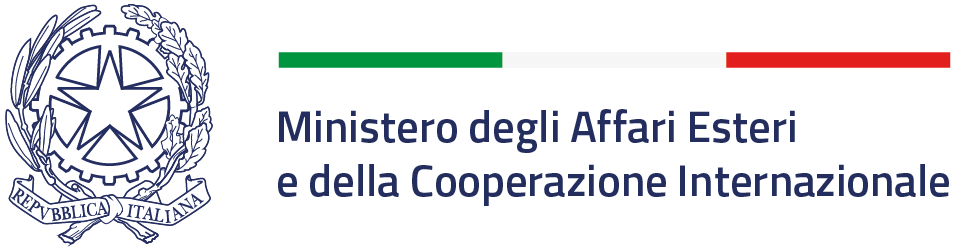
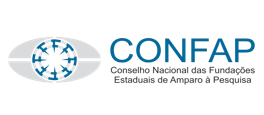
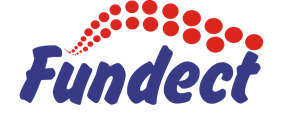